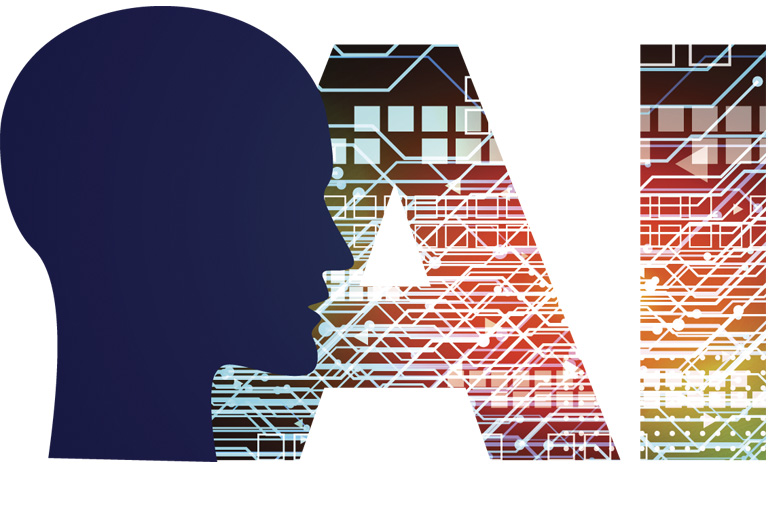
Digital transformation requires an automated and machine-assisted approach
Digital business transformation is forcing IT organizations to reconsider how to ensure infrastructure and application performance. Speed, scale, and complexity brought on by multi-cloud infrastructure and digitization stress traditional rules-based performance monitoring and management. AIOps applies machine learning and advanced analytics techniques to identify patterns in monitoring, service desk, and automation data that is so vast it is otherwise beyond human comprehension.
AIOps platforms are software systems that combine big data and AI or machine learning functionality to enhance and partially replace a broad range of IT operations processes and tasks, including availability and performance monitoring, event correlation and analysis, IT service management, and automation.
Source: Gartner Market Guide for AIOps Platforms Published: 03 August 2017 ID: G00322184
Key elements of an AIOps approach
Implementing an AIOps approach goes beyond getting better analytics for existing data. Building the basis for a machine learning system that will yield continuous insights requires the below 4 key elements.
Open data access
Open data access including multiple, consumable sources of historical and streaming IT data of the four key elements, the most critical is open data access. Core IT will always have multiple technologies and systems of record from different vendors. These will also vary across IT disciplines. Freeing data from its organizational silos for big data aggregation and analysis is perhaps the most difficult challenge facing IT teams trying to implement AIOps.
An effective AIOps platform must have a data schema that can consume data from a variety of IT sources, and structure, tag, and organize it to be useful for consistent and repeatable analysis.
Big data scale and speed
A big data platform that can support real-time visualization and deep queries Digital transformation means high volumes of rapidly changing IT data. Traditional relational data warehouses are neither scalable nor responsive enough to support the quantity and speed of digital data. Analysis needs to take place in real-time on data as it comes in – not only offline when resources are available.
An AIOps big data platform must also support responsive ad-hoc data exploration and deep queries. Big data technologies, originally created to handle large data lakes from data warehouses, have rapidly evolved into not just scalable but responsive data manipulation engines that can meet the needs of AIOps. AIOps represents the unification of deep data research and online, real-time analytics to elevate IT decision making.
Machine learning
Machine learning that refines the algorithms based on the data without human intervention, AIOps enables IT to move from rule-based, human management of analysis to machine-assisted analysis and machine learning systems. This is required not only because of limits to the amount and complexity of analysis human agents can achieve, but also to enable a level of change adaptation that hasn’t been possible.
IT analytics is ultimately about pattern matching. IT systems, users and ecosystems exhibit behaviors and relationships that can point to root causes, isolate issues, and indicate future problems. Machine learning applies the computational power and speed of machines to the discovery and correlation of patterns in IT data. It does this more and faster than human agents and dynamically changes the algorithms used by analytics based on changes in the data.
AIOps and automation
Analytical algorithms that yield automated IT insights on IT data for IT purposes, AIOps connects and drives automation in the hyper-complex, multi-source cloud environment. Delivering machine-assisted analytics at scale on high volumes of digital IT data is useless if the outcomes still require human intervention. AIOps can generate workflows and measure the effects of those processes, feeding the results back into the system as data to be analyzed and learned from. Additionally, AIOps should be applied by the system automatically based on the data, without the need for user intervention and decision.
The democracy of data
IT needs to focus on enabling digital business transformation. It should not have to have dedicated data specialists or scientists to feed and create analytics systems, nor hire or develop data analysis skills in IT personnel. AIOps leverages machines to do this work without the need for specialized resources. The output of analysis can be consumed and customized by anyone in the IT organization and can be easily extended to partners across the business.
- Open data access
- Big data scale and speed
- Machine learning
- AIOps and automation
- The democracy of data
Open data access
Open data access including multiple, consumable sources of historical and streaming IT data of the four key elements, the most critical is open data access. Core IT will always have multiple technologies and systems of record from different vendors. These will also vary across IT disciplines. Freeing data from its organizational silos for big data aggregation and analysis is perhaps the most difficult challenge facing IT teams trying to implement AIOps.
An effective AIOps platform must have a data schema that can consume data from a variety of IT sources, and structure, tag, and organize it to be useful for consistent and repeatable analysis.
Big data scale and speed
A big data platform that can support real-time visualization and deep queries Digital transformation means high volumes of rapidly changing IT data. Traditional relational data warehouses are neither scalable nor responsive enough to support the quantity and speed of digital data. Analysis needs to take place in real-time on data as it comes in – not only offline when resources are available.
An AIOps big data platform must also support responsive ad-hoc data exploration and deep queries. Big data technologies, originally created to handle large data lakes from data warehouses, have rapidly evolved into not just scalable but responsive data manipulation engines that can meet the needs of AIOps. AIOps represents the unification of deep data research and online, real-time analytics to elevate IT decision making.
Machine learning
Machine learning that refines the algorithms based on the data without human intervention, AIOps enables IT to move from rule-based, human management of analysis to machine-assisted analysis and machine learning systems. This is required not only because of limits to the amount and complexity of analysis human agents can achieve, but also to enable a level of change adaptation that hasn’t been possible.
IT analytics is ultimately about pattern matching. IT systems, users and ecosystems exhibit behaviors and relationships that can point to root causes, isolate issues, and indicate future problems. Machine learning applies the computational power and speed of machines to the discovery and correlation of patterns in IT data. It does this more and faster than human agents and dynamically changes the algorithms used by analytics based on changes in the data.
AIOps and automation
Analytical algorithms that yield automated IT insights on IT data for IT purposes, AIOps connects and drives automation in the hyper-complex, multi-source cloud environment. Delivering machine-assisted analytics at scale on high volumes of digital IT data is useless if the outcomes still require human intervention. AIOps can generate workflows and measure the effects of those processes, feeding the results back into the system as data to be analyzed and learned from. Additionally, AIOps should be applied by the system automatically based on the data, without the need for user intervention and decision.
The democracy of data
IT needs to focus on enabling digital business transformation. It should not have to have dedicated data specialists or scientists to feed and create analytics systems, nor hire or develop data analysis skills in IT personnel. AIOps leverages machines to do this work without the need for specialized resources. The output of analysis can be consumed and customized by anyone in the IT organization and can be easily extended to partners across the business.
Solutions for AIOps
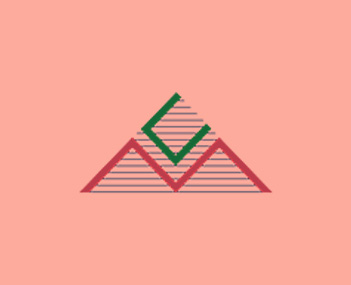
TrueSight
Portfolio of products offering unprecedented visibility into the performance, security and usage of IT resources.
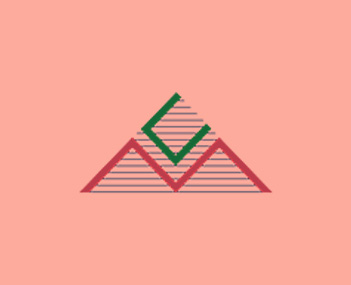
BMC Helix ITSM
Predictive IT service management for modern enterprise.
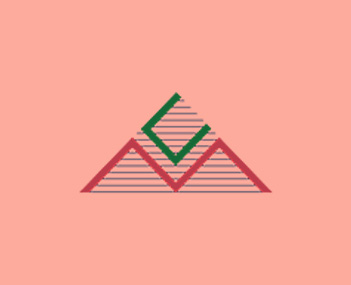
TrueSight Orchestration
Coordinate workflows across applications, platforms and tools to automate critical IT processes.